Forest Fire Probability Mapping in Eastern Serbia: Logistic Regression versus Random Forest Method
Само за регистроване кориснике
2021
Аутори
Milanović, Slobodan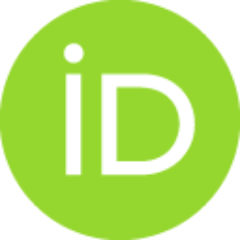
Marković, Nenad
Pamučar, Dragan
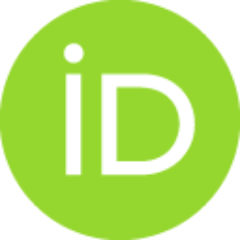
Gigović, Ljubomir
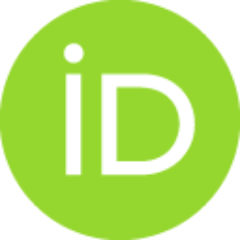
Kostić, Pavle
Milanović, Slađan D.
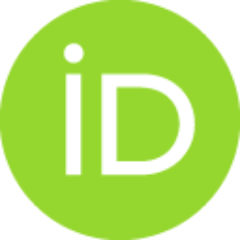
Чланак у часопису (Објављена верзија)
Метаподаци
Приказ свих података о документуАпстракт
Forest fire risk has increased globally during the previous decades. The Mediterranean region is traditionally the most at risk in Europe, but continental countries like Serbia have experienced significant economic and ecological losses due to forest fires. To prevent damage to forests and infrastructure, alongside other societal losses, it is necessary to create an effective protection system against fire, which minimizes the harmful effects. Forest fire probability mapping, as one of the basic tools in risk management, allows the allocation of resources for fire suppression, within a fire season, from zones with a lower risk to those under higher threat. Logistic regression (LR) has been used as a standard procedure in forest fire probability mapping, but in the last decade, machine learning methods such as fandom forest (RF) have become more frequent. The main goals in this study were to (i) determine the main explanatory variables for forest fire occurrence for both models, LR and ...RF, and (ii) map the probability of forest fire occurrence in Eastern Serbia based on LR and RF. The most important variable was drought code, followed by different anthropogenic features depending on the type of the model. The RF models demonstrated better overall predictive ability than LR models. The map produced may increase firefighting efficiency due to the early detection of forest fire and enable resources to be allocated in the eastern part of Serbia, which covers more than one-third of the country's area.
Кључне речи:
variable importance / prediction accuracy / occurrence of forest fire / machine learningИзвор:
Forests, 2021, 12, 1Финансирање / пројекти:
- Ministry of Agriculture, Forestry and Water Management of the Republic of Serbia-Forest Directorate [401-00-1713/2019-10]
- Министарство науке, технолошког развоја и иновација Републике Србије, институционално финансирање - 200015 (Универзитет у Београду, Институт за медицинска истраживања) (RS-MESTD-inst-2020-200015)
DOI: 10.3390/f12010005
ISSN: 1999-4907
WoS: 000610210900001
Scopus: 2-s2.0-85099476651
Институција/група
Šumarski fakultetTY - JOUR AU - Milanović, Slobodan AU - Marković, Nenad AU - Pamučar, Dragan AU - Gigović, Ljubomir AU - Kostić, Pavle AU - Milanović, Slađan D. PY - 2021 UR - https://omorika.sfb.bg.ac.rs/handle/123456789/1211 AB - Forest fire risk has increased globally during the previous decades. The Mediterranean region is traditionally the most at risk in Europe, but continental countries like Serbia have experienced significant economic and ecological losses due to forest fires. To prevent damage to forests and infrastructure, alongside other societal losses, it is necessary to create an effective protection system against fire, which minimizes the harmful effects. Forest fire probability mapping, as one of the basic tools in risk management, allows the allocation of resources for fire suppression, within a fire season, from zones with a lower risk to those under higher threat. Logistic regression (LR) has been used as a standard procedure in forest fire probability mapping, but in the last decade, machine learning methods such as fandom forest (RF) have become more frequent. The main goals in this study were to (i) determine the main explanatory variables for forest fire occurrence for both models, LR and RF, and (ii) map the probability of forest fire occurrence in Eastern Serbia based on LR and RF. The most important variable was drought code, followed by different anthropogenic features depending on the type of the model. The RF models demonstrated better overall predictive ability than LR models. The map produced may increase firefighting efficiency due to the early detection of forest fire and enable resources to be allocated in the eastern part of Serbia, which covers more than one-third of the country's area. T2 - Forests T1 - Forest Fire Probability Mapping in Eastern Serbia: Logistic Regression versus Random Forest Method IS - 1 VL - 12 DO - 10.3390/f12010005 UR - conv_1525 ER -
@article{ author = "Milanović, Slobodan and Marković, Nenad and Pamučar, Dragan and Gigović, Ljubomir and Kostić, Pavle and Milanović, Slađan D.", year = "2021", abstract = "Forest fire risk has increased globally during the previous decades. The Mediterranean region is traditionally the most at risk in Europe, but continental countries like Serbia have experienced significant economic and ecological losses due to forest fires. To prevent damage to forests and infrastructure, alongside other societal losses, it is necessary to create an effective protection system against fire, which minimizes the harmful effects. Forest fire probability mapping, as one of the basic tools in risk management, allows the allocation of resources for fire suppression, within a fire season, from zones with a lower risk to those under higher threat. Logistic regression (LR) has been used as a standard procedure in forest fire probability mapping, but in the last decade, machine learning methods such as fandom forest (RF) have become more frequent. The main goals in this study were to (i) determine the main explanatory variables for forest fire occurrence for both models, LR and RF, and (ii) map the probability of forest fire occurrence in Eastern Serbia based on LR and RF. The most important variable was drought code, followed by different anthropogenic features depending on the type of the model. The RF models demonstrated better overall predictive ability than LR models. The map produced may increase firefighting efficiency due to the early detection of forest fire and enable resources to be allocated in the eastern part of Serbia, which covers more than one-third of the country's area.", journal = "Forests", title = "Forest Fire Probability Mapping in Eastern Serbia: Logistic Regression versus Random Forest Method", number = "1", volume = "12", doi = "10.3390/f12010005", url = "conv_1525" }
Milanović, S., Marković, N., Pamučar, D., Gigović, L., Kostić, P.,& Milanović, S. D.. (2021). Forest Fire Probability Mapping in Eastern Serbia: Logistic Regression versus Random Forest Method. in Forests, 12(1). https://doi.org/10.3390/f12010005 conv_1525
Milanović S, Marković N, Pamučar D, Gigović L, Kostić P, Milanović SD. Forest Fire Probability Mapping in Eastern Serbia: Logistic Regression versus Random Forest Method. in Forests. 2021;12(1). doi:10.3390/f12010005 conv_1525 .
Milanović, Slobodan, Marković, Nenad, Pamučar, Dragan, Gigović, Ljubomir, Kostić, Pavle, Milanović, Slađan D., "Forest Fire Probability Mapping in Eastern Serbia: Logistic Regression versus Random Forest Method" in Forests, 12, no. 1 (2021), https://doi.org/10.3390/f12010005 ., conv_1525 .